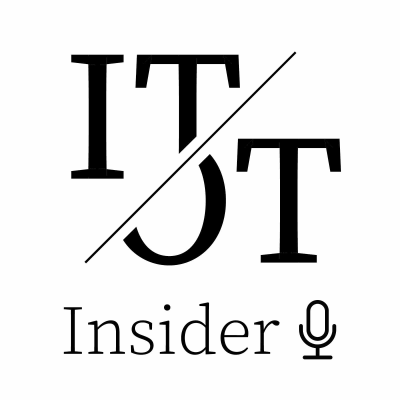
The IT/OT Insider Podcast - Pioneers & Pathfinders
Podcast door By David Ariens and Willem van Lammeren
Tijdelijke aanbieding
3 maanden voor € 1,00
Daarna € 9,99 / maandElk moment opzegbaar.
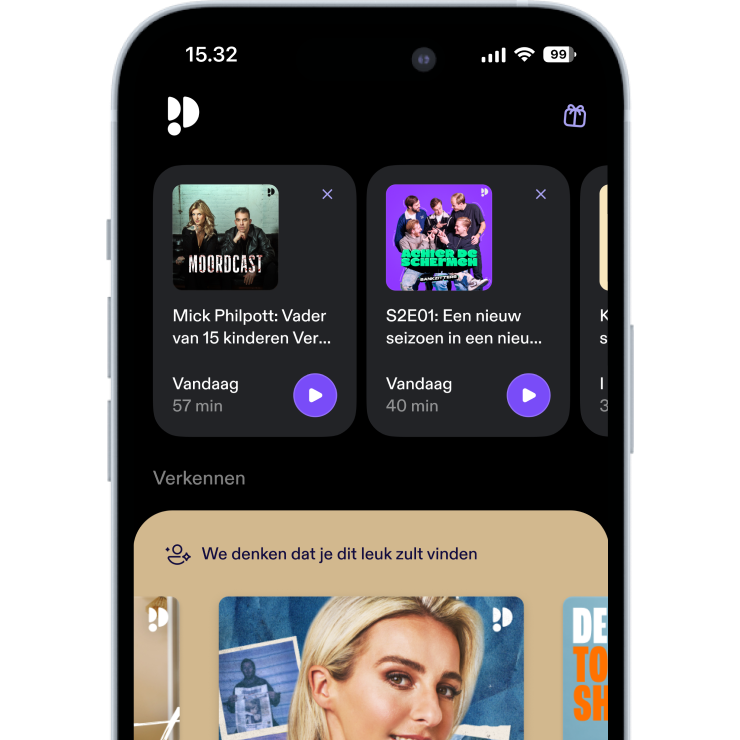
Meer dan 1 miljoen luisteraars
Je zult van Podimo houden en je bent niet de enige
Rated 4.7 in the App Store
Over The IT/OT Insider Podcast - Pioneers & Pathfinders
How can we really digitalize our Industry? Join us as we navigate through the innovations and challenges shaping the future of manufacturing and critical infrastructure. From insightful interviews with industry leaders to deep dives into transformative technologies, this podcast is your guide to understanding the digital revolution at the heart of the physical world. We talk about IT/OT Convergence and focus on People & Culture, not on the Buzzwords. To support the transformation, we discover which Technologies (AI! Cloud! IIoT!) can enable this transition. itotinsider.substack.com
Alle afleveringen
34 afleveringen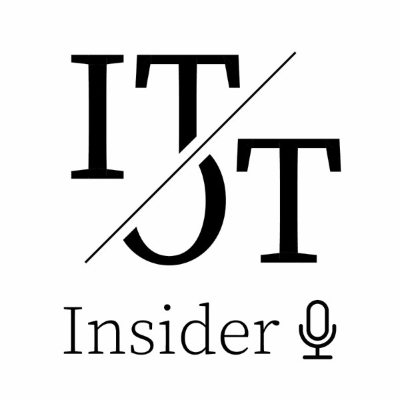
In this episode of the IT/OT Insider Podcast, we welcome someone who doesn’t come from cloud platforms, data infrastructure, or connectivity layers. Instead, he brings something equally vital: operational wisdom. Raf Swinnen has spent his career inside factories. From Procter & Gamble to Kellogg's, and later Danone, Raf worked at the intersection of operations and transformation, guiding teams through continuous improvement and later, digital initiatives. What makes his perspective especially valuable? It’s grounded in Lean thinking. Not as a buzzword, but as a real discipline. One that requires a sharp understanding of processes, a respect for people on the floor, and a strong filter for what actually adds value. From Line Leader to Digital Change Agent Raf didn’t start in digital. He started on the floor: managing lines, people, safety, and performance. That experience shaped how he sees digital transformation today: as something that should support operations, not get in the way of them. At Danone, he led digital initiatives at the Rotselaar site (Belgium). The job wasn’t to implement more dashboards. It was to help teams use data to drive better decisions, without losing sight of the fundamentals. “Tech to the Back” — What Digital Should Learn from Lean One of the most powerful takeaways from this episode is Raf’s principle of “Tech to the back.” “Digital solutions should not be front and center. People and processes should be. Tech should follow.” This is a strong antidote to the over-designed, solution-first approaches that often flood the industrial space. According to Raf, the biggest risk in digital projects isn’t the technology — it’s losing the problem along the way. Three C’s: Connect, Collaborate, and Coherence As part of his work with leadership teams, Raf often introduces what he calls the 3 C’s: * Clarity – Where are we going, and why? * Consistency – Are we reinforcing the same messages and systems? * Coherence – Do our tools, apps, and data work together? These are not slogans, they are essential behaviors for any transformation to stick. They also align closely with how we designed the ITOT.Academy [https://itot.academy], where cross-role learning and shared frameworks are front and center. One of Raf’s biggest contributions came through how he structured teams. In a newly created role as Digital Program Manager, he pulled in both IT and OT voices and even shifted reporting lines to foster true collaboration. He didn’t look for tech wizards. He looked for people with enthusiasm. People who wanted to make a difference. These became his digital ambassadors, key voices from every shift, every team. “When the night shift speaks up, you listen. They see the edge cases nobody else does.” Case Examples: Real Change Starts Small Raf shared stories from his time at Danone, Kellogg’s, and P&G, where transformation didn’t come from big declarations — but from small, disciplined steps. At one plant, it was about helping teams make better use of their shift handovers.At another, it meant cleaning up data before launching another round of training.At Danone, the challenge was scaling good ideas without flattening local ownership. “Digital without context is noise. The real challenge is creating relevance at the point of use.” Digital with Discipline Raf’s story is a reminder that digital transformation doesn’t start with technology, it starts with understanding the process. Listening to the people who run it, and designing with clarity and purpose. Whether it's Lean principles, cultural alignment, or simply asking better questions, his approach keeps the focus where it matters: on solving real problems in practical ways. In a time when industrial tech is advancing fast and buzzwords multiply by the day, it’s refreshing to hear someone say: let’s not forget why we’re doing this in the first place. If you’re working in digital, operations, or somewhere in between, this episode is a pause-and-reflect moment. And maybe also a nudge: to push tech to the back, and put people and purpose out front. Disclaimer: The views and opinions expressed in this interview are those of the interviewee and do not necessarily reflect the official policy or position of The IT/OT Insider. This content is provided for informational purposes only and should not be seen as an endorsement by The IT/OT Insider of any products, services, or strategies discussed. We encourage our readers and listeners to consider the information presented and make their own informed decisions. This is a public episode. If you would like to discuss this with other subscribers or get access to bonus episodes, visit itotinsider.substack.com [https://itotinsider.substack.com?utm_medium=podcast&utm_campaign=CTA_1]
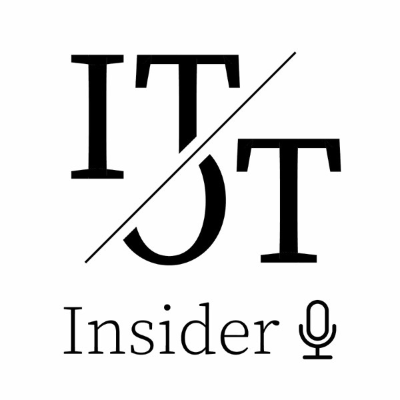
📣 Quick note before we dive into all things open source: In our last episode [https://itotinsider.substack.com/p/were-launching-the-itotacademy-and], we announced the launch of the https://itot.academyITOT.Academy [https://itot.academy]: a live on-line learning experience for professionals navigating the complex world of IT/OT collaboration. Our early bird seats are filling up fast. If you’re serious about gaining practical skills (not just theory), now’s the time to secure your spot. Don’t wait too long, the first cohorts start on August 29 and September 5 (each cohort consists of six 2 hour sessions and you receive all recordings). 👉 Full training program and registration via ITOT.Academy [https://itot.academy] In this episode of the IT/OT Insider Podcast, we sit down with Alexander Krüger, co-founder and CEO of United Manufacturing Hub [https://www.umh.app/] (UMH), to talk about something that’s both old and revolutionary in the industrial world: open source software. This isn’t about hobby projects or side experiments. It’s about why open source is playing an increasingly important role in how factories move data, scale operations, and reduce vendor lock-in. Alexander brings both a technical and business perspective and shares what happens when a mechanical engineer dives deep into the world of cloud-native data infrastructure. Not all Open Source is created equal Most industrial companies still equate reliability with paying a vendor and signing a service-level agreement. But Alexander challenges that mindset. His team originally built UMH because they were frustrated with how hard it was to try, test, and scale traditional industrial software. “We just wanted to get data from A to B in a factory, but realized that problem isn’t really solved yet. So we made it open source.” Alexander is quick to point out that choosing open source doesn’t automatically mean less risk, but it does mean different trade-offs. Key factors include: * Licensing clarity * Community health (Is it maintained? Is it active?) * Governance (Who controls the roadmap? What happens if they change direction?) He even brings up the infamous example of vendors repackaging tools like Node-RED under different names, then charging for them without giving proper credit (or worse, shipping outdated versions). “If you’re already bundling open source into your software, why not be honest about it?” What about reliability? If you’re an OT leader, you might still worry: who do I call at 2 a.m. when something breaks? Alexander’s answer: you should be asking that question about any software, open or proprietary. Because often, what fails isn’t the software itself, it’s the integrations someone built in a rush, or the one engineer who knew how things worked and then left the company. With open source, there’s at least transparency, control, and the ability to maintain continuity. You’re not locked out of your own systems. The Human Side: The rise of the hybrid engineer One of the most interesting parts of the conversation was about who will make this all work. Alexander sees a new kind of engineer emerging: someone with a background in OT, but who enjoys learning IT concepts, tinkering with Docker, and embracing DevOps practices. “We’re looking for people who used to live in TIA Portal but now run state of the art home automation in their free time.” This isn’t about turning everyone into a software developer. But it is about building a culture where people are open to learning from both sides and using modern ways of working and new tools to solve old problems. Stay Tuned for More! Subscribe to our podcast and blog to stay updated on the latest trends in Industrial Data, AI, and IT/OT convergence. 🚀 See you in the next episode! Youtube: https://www.youtube.com/@TheITOTInsider [https://www.youtube.com/@TheITOTInsider] Apple Podcasts: Spotify Podcasts: Disclaimer: The views and opinions expressed in this interview are those of the interviewee and do not necessarily reflect the official policy or position of The IT/OT Insider. This content is provided for informational purposes only and should not be seen as an endorsement by The IT/OT Insider of any products, services, or strategies discussed. We encourage our readers and listeners to consider the information presented and make their own informed decisions. This is a public episode. If you would like to discuss this with other subscribers or get access to bonus episodes, visit itotinsider.substack.com [https://itotinsider.substack.com?utm_medium=podcast&utm_campaign=CTA_1]
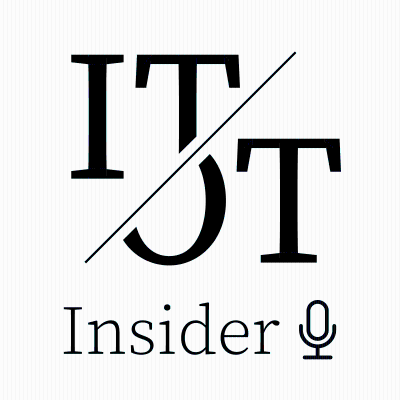
Discover the program and claim your seat here: https://itot.academy [https://itot.academy] 🎙️ In this special episode of the IT/OT Insider Podcast, David and Willem officially announce the launch of the ITOT.Academy [https://itot.academy]! After years of conversations with IT/OT professionals, consultants, and technology vendors, one thing became clear: there’s a huge need for practical, vendor-neutral education to help people work together across IT and OT boundaries. The ITOT.Academy [https://itot.academy] is designed to fill that gap. What you’ll learn in this episode:Why we created the AcademyWho it's for: OT teams, IT teams, consultants, vendorsThe structure of the program: short, live, interactive sessionsWhy it's not about convergence but collaborationWhen the first groups will startHow to sign up and join the first cohorts🚀 Learn more and sign up at https://itot.academy [https://itot.academy] 🎧 Subscribe for more honest conversations on bridging IT and OT. Chapters 00:00 Introduction to ITOT Academy 01:38 Feedback from Subscribers 03:47 Target Audience for Training 07:24 Training Format and Structure 11:19 Core Concepts of the Training 13:32 Interactive Sessions and Wrap-Up 14:37 Launch Details and Closing Remarks This is a public episode. If you would like to discuss this with other subscribers or get access to bonus episodes, visit itotinsider.substack.com [https://itotinsider.substack.com?utm_medium=podcast&utm_campaign=CTA_1]
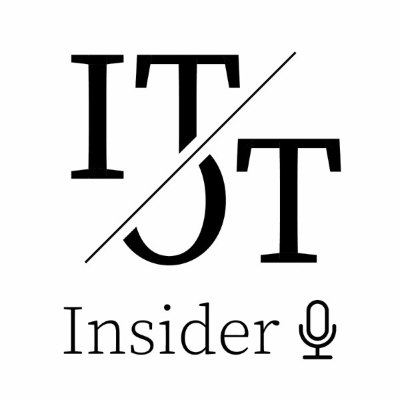
It is episode 31 and we’re finally tackling a topic that somehow hadn’t made the spotlight yet: IoT. And we couldn’t have asked for two better guests to help us dive into it: Olivier Bloch and Ryan Kershaw. This is not your usual shiny, buzzword-heavy conversation about the Internet of Things. Olivier and Ryan bring decades of hands-on experience from both sides of the IT/OT divide: Olivier from embedded systems, developer tooling, and cloud platforms, Ryan from the shop floor, instrumentation, and operational systems. Together, they’re building bridges where others see walls. IoT 101 Olivier kicks things off with a useful reset: "IoT is anything that has compute and isn’t a traditional computer. But more importantly, it’s the layer that lets these devices contribute to a bigger system: by sharing data, receiving commands, and acting in context." Olivier has seen IoT evolve from standalone embedded devices to edge-connected machines, then cloud-managed fleets, and now towards context-aware, autonomous systems that require real-time decision-making. Ryan, meanwhile, brings us back to basics: "When I started, a pH sensor gave you one number. Now, it gives you twelve: pH, temperature, calibration life, glass resistance... The challenge isn’t getting the data. It’s knowing what to do with it." Infrastructure Convergence: The Myth of the One-Size-Fits-All Platform We asked the obvious question: after all these years, why hasn’t “one platform to rule them all” emerged for IoT? Olivier’s take is straightforward: "All the LEGO bricks are out there. The hard part is assembling them for your specific need. Most platforms try to do too much or don’t understand the OT context." You can connect anything these days. The real question is: should you? Start small, solve a problem, and build trust from there. Why Firewalls are no longer enough Another highlight: their views on security and zero trust in industrial environments. Olivier and Ryan both agree: the old-school "big fat firewall" between IT and OT isn’t enough. "You’re not just defending a perimeter anymore. You need to assume compromise and secure each device, user, and transaction individually." So what is Zero Trust, exactly? It’s a cybersecurity model that assumes no device, user, or system should be automatically trusted, whether it’s inside or outside the network perimeter. Instead of relying on a single barrier like a firewall, Zero Trust requires continuous verification of every request, with fine-grained access control, identity validation, and least-privilege permissions. It’s a mindset shift: never trust, always verify. They also emphasize that zero trust doesn’t mean "connect everything." Sometimes the best security strategy is to not connect at all, or to use non-intrusive sensors instead of modifying legacy equipment. Brownfield vs. Greenfield: Two different journeys When it comes to industrial IoT, where you start has everything to do with what you can do. Greenfield projects, like new plants or production lines, offer a clean slate. You can design the network architecture from the ground up, choose modern protocols like MQTT, and enforce consistent naming and data modeling across all assets. This kind of environment makes it much easier to build a scalable, reliable IoT system with fewer compromises. Brownfield environments are more common and significantly more complex. These sites are full of legacy PLCs, outdated SCADA systems, and equipment that was never meant to connect to the internet. The challenge is not just technical. It's also cultural, operational, and deeply embedded in the way people work. "In brownfield, you can’t rip and replace. You have to layer on carefully, respecting what works while slowly introducing what’s new," said Ryan. Olivier added that in either case, the mistake is the same: moving too fast without thinking ahead. "The mistake people make in brownfield is to start too scrappy. It’s tempting to just hack something together. But you’ll regret it later when you need to scale or secure it." Their advice is simple: Even if you're solving one problem, design like you will solve five. That means using structured data models, modular components, and interfaces that can evolve. Final Thoughts This episode was a first deep dive into real-world IoT—not just the buzzwords, but the architecture, trade-offs, and decision-making behind building modern industrial systems. From embedded beginnings to UNS ambitions, Thing-Zero is showing that the future of IoT isn’t about more tech. It’s about making better choices, backed by cross-disciplinary teams who understand both shop floor realities and enterprise demands. To learn more, visit thing-zero.com [https://thing-zero.com] and check out Olivier’s YouTube channel “The IoT Show [https://www.youtube.com/channel/UC4y05njclZL_WaQZ_xgnAgQ]” for insightful and developer-focused content. Stay Tuned for More! Subscribe to our podcast and blog to stay updated on the latest trends in Industrial Data, AI, and IT/OT convergence. 🚀 See you in the next episode! Youtube: https://www.youtube.com/@TheITOTInsider [https://www.youtube.com/@TheITOTInsider] Apple Podcasts: Spotify Podcasts: Disclaimer: The views and opinions expressed in this interview are those of the interviewee and do not necessarily reflect the official policy or position of The IT/OT Insider. This content is provided for informational purposes only and should not be seen as an endorsement by The IT/OT Insider of any products, services, or strategies discussed. We encourage our readers and listeners to consider the information presented and make their own informed decisions. This is a public episode. If you would like to discuss this with other subscribers or get access to bonus episodes, visit itotinsider.substack.com [https://itotinsider.substack.com?utm_medium=podcast&utm_campaign=CTA_1]
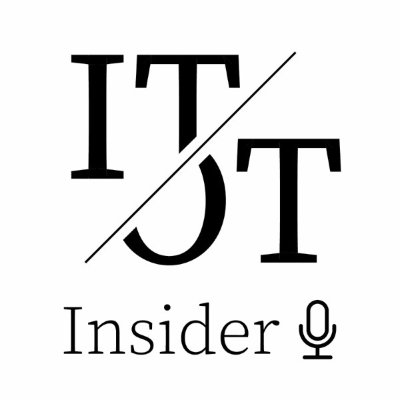
Today, we have the pleasure of speaking with Nikki Gonzales, Director of Business Development at Weintek USA, co-founder of the Automation Ladies podcast, and co-organizer of OT SCADA CON—a conference focused on the gritty, real-world challenges of industrial automation. Unlike many of our guests who often come from cloud-first, data-driven digitalization backgrounds, Nikki brings a refreshing and much-needed OT floor-level perspective. Her world is HMI screens, SCADA systems, manufacturers, machine builders, and the hard truths about where industry transformation actually stands today. What’s an HMI and Why Does It Matter? In Nikki’s words, an HMI is: "The bridge between the operator, the machine, and the greater plant network." It’s often misunderstood as just a touchscreen replacement for buttons—but Nikki highlights that a modern HMI can do much more: * Act as a gateway between isolated machines and plant-level networks. * Enable remote access, alarm management, and contextual data sharing. * Help standardize connectivity in mixed-vendor environments. The HMI is often the first step in connecting legacy equipment to broader digital initiatives. Industry 3.0 vs. Industry 4.0: Ground Reality Check While the industry buzzes with Industry 4.0 (and 5.0 🙃) concepts, Nikki’s view from the field is sobering: "Most small manufacturers are still living in Industry 3.0—or earlier. They have mixed equipment, proprietary protocols, and minimal digitalization." For the small manufacturers Nikki works with, transformation isn't about launching huge digital projects. It’s about taking incremental steps: * Upgrading a handful of sensors. * Introducing remote monitoring. * Standardizing alarm management. * Gradually building operational visibility. "Transformation for small companies isn’t about fancy AI. It’s about survival—staying competitive, keeping workers, and staying in business." With labor shortages, supply chain pressures, and rising cybersecurity threats, smaller manufacturers must adapt—but they have to do it in a way that is affordable, modular, and low-risk. UNS, SCADA, and the State of Connectivity Nikki also touched on how concepts like UNS (Unified Namespace) are being discussed: "Everyone talks about UNS and cloud-first strategies. But in reality, most plants still have islands of automation. They have to bridge old PLCs, proprietary protocols, and aging SCADA systems first." While UNS represents a desirable goal—a real-time, unified data model accessible across the enterprise—many manufacturers are years (or even decades) away from making that a reality without significant groundwork first. In this world, HMI upgrades, standardized communication protocols (like MQTT), and targeted SCADA modernization become the critical building blocks. The Human Challenge: Culture and Workforce Beyond the technology, Nikki highlighted the human side of transformation: * Younger generations aren't attracted to repetitive, low-tech manufacturing jobs. * Manual, isolated processes make hiring and retention even harder. * Manufacturers must rethink how technology supports not just efficiency, but employee satisfaction. The future of manufacturing depends not just on smarter machines—but on designing operations that attract and empower the next generation of workers. Organizing a Conference from Scratch: OT SCADA CON Before wrapping up, we asked Nikki about organizing OT SCADA CON [https://www.otscada.com/]. "You need a little naivety, a lot of persistence, and the right partners. We jumped first, then figured out how to build the plane on the way down." OT SCADA CON is designed by practitioners for practitioners—short technical sessions, no vendor pitches, no buzzword bingo. Just real, practical advice for the engineers, integrators, and plant technicians who make industrial operations work. Final Thoughts In a world obsessed with the future, Nikki reminds us: You can't build Industry 4.0 without first fixing Industry 3.0. And fixing it starts with respecting the complexity, valuing the small steps, and supporting the people on the ground who keep manufacturing running. If you want to learn more about Nikki’s work, visit https://automationladies.io/automationladies.io [https://automationladies.io/] and check out OT SCADA CON [https://www.otscada.com/], taking place July 23–25, 2025. Stay Tuned for More! Subscribe to our podcast and blog to stay updated on the latest trends in Industrial Data, AI, and IT/OT convergence. 🚀 See you in the next episode! Youtube: https://www.youtube.com/@TheITOTInsider [https://www.youtube.com/@TheITOTInsider] Apple Podcasts: Spotify Podcasts: Disclaimer: The views and opinions expressed in this interview are those of the interviewee and do not necessarily reflect the official policy or position of The IT/OT Insider. This content is provided for informational purposes only and should not be seen as an endorsement by The IT/OT Insider of any products, services, or strategies discussed. We encourage our readers and listeners to consider the information presented and make their own informed decisions. This is a public episode. If you would like to discuss this with other subscribers or get access to bonus episodes, visit itotinsider.substack.com [https://itotinsider.substack.com?utm_medium=podcast&utm_campaign=CTA_1]
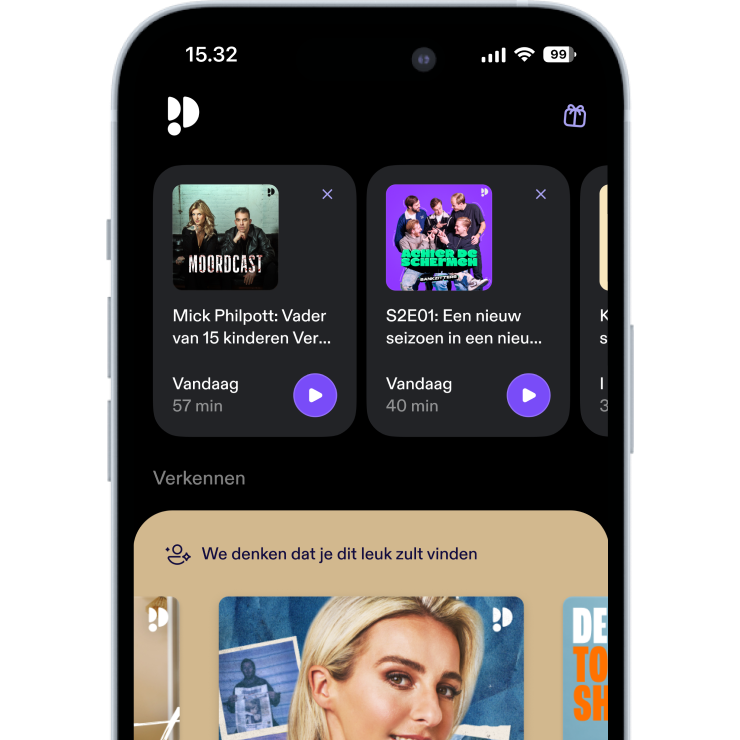
Rated 4.7 in the App Store
Tijdelijke aanbieding
3 maanden voor € 1,00
Daarna € 9,99 / maandElk moment opzegbaar.
Exclusieve podcasts
Advertentievrij
Gratis podcasts
Luisterboeken
20 uur / maand