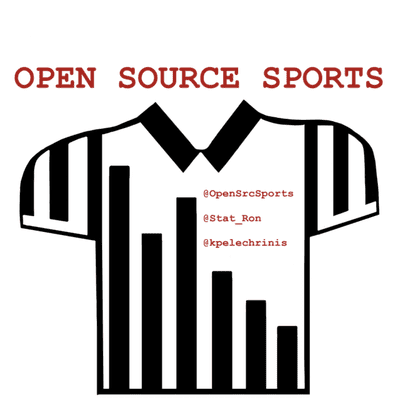
Open Source Sports
Podcast de Ron Yurko
Ron Yurko and Kostas Pelechrinis host the 'Open Source Sports' podcast to serve as a public reading group for discussing the latest research in sports analytics. Each episode focuses on a single paper featuring authors as guests, with discussions about the statistical methodology, relevance and future directions of the research.
Empieza 30 días de prueba
4,99 € / mes después de la prueba.Cancela cuando quieras.
Todos los episodios
12 episodios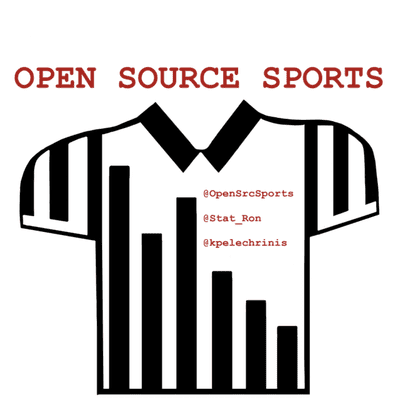
In this episode we talk to Stephanie Kovalchik [https://twitter.com/StatsOnTheT] about her paper 'A Statistical Model of Serve Return Impact Patterns in Professional Tennis' [https://arxiv.org/abs/2202.00583] (co-authored with Jim Albert). Stephanie is a Staff Data Scientist at Zelus Analytics [https://zelusanalytics.com/], where she works on advanced performance valuation for multiple pro sports. Before joining Zelus, Stephanie led data science innovation for the Game Insight Group of Tennis Australia [https://www.tennis.com.au/], building first-of-a-kind metrics and real-time applications with tracking data. Stephanie is the founder of the tennis analytics blog "On the T" [http://on-the-t.com/] and tweets @StatsOnTheT [https://twitter.com/StatsOnTheT]. For additional references mentioned in the show: * ATP Tour Second Screen [https://www.atptour.com/en/stats/second-screen/archive/2022/404/MS001] * Stephanie's article in Harvard Data Science Review: Why Tennis Is Still Not Ready to Play Moneyball [https://hdsr.mitpress.mit.edu/pub/uy0zl4i1/release/4] * Grand Slam R package: courtvisionr [https://github.com/skoval/courtvisionr] * Stephanie's GitHub with various resources for accessing tennis data: https://github.com/skoval [https://github.com/skoval] * Stan tutorials: https://mc-stan.org/users/documentation/tutorials [https://mc-stan.org/users/documentation/tutorials] * Register now for the Carnegie Mellon Sports Analytics Conference: https://www.stat.cmu.edu/cmsac/conference/2022/ [https://www.stat.cmu.edu/cmsac/conference/2022/] * Check out the Big Data Derby [https://www.kaggle.com/competitions/big-data-derby-2022/overview] now on Kaggle
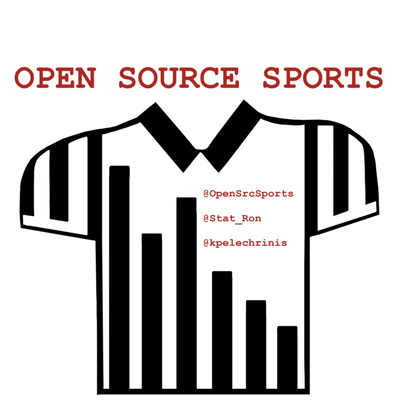
We discuss True Shot Charts [https://papers.ssrn.com/sol3/papers.cfm?abstract_id=4138586] with Syracuse University Professors Justin Ehrlich [https://falk.syr.edu/people/ehrlich-justin/] and Shane Sanders [https://falk.syr.edu/people/sandersshane/]. For references mentioned in the show: * BigDataBall [https://www.bigdataball.com/] * StatMuse [https://www.statmuse.com/nba] * Positive Residual [https://www.positiveresidual.com/] - True Shooting Charts [https://www.positiveresidual.com/shiny/true-shooting-charts/]
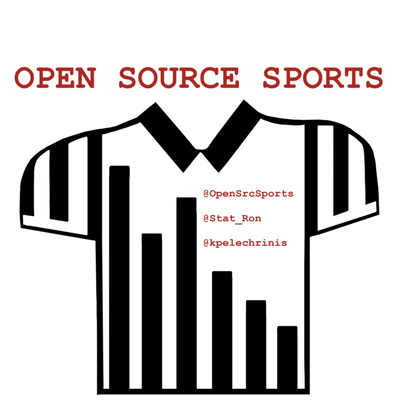
We discuss An Examination of Olympic Sport Climbing Competition Format and Scoring System [https://jds-online.org/journal/JDS/article/1273/info] with Quang Nguyen [https://qntkhvn.netlify.app/] (@qntkhvn [https://twitter.com/qntkhvn]). This paper won the Carnegie Mellon Sports Analytics Conference Reproducible Research Competition in November 2021 [https://www.stat.cmu.edu/cmsac/conference/2021/]. [http://stat.cmu.edu/cmsac/conference/2020/] Quang Nguyen completed his Master of Science in Applied Statistics at Loyola University Chicago [https://www.luc.edu/datascience/] in 2021. He recently spent the Spring 2022 semester working as an instructor in the Dept of Mathematics and Statistics at Loyola. Quang previously completed his undergraduate degree in Mathematics and Data Science at Wittenberg University in Springfield, Ohio. Quang's current interests include statistics in sports, data science, statistics and data science education, and reproducibility. He is a die-hard supporter of Manchester United F.C. of the English Premier League. And last but not least, Quang is excited to join the Dept of Statistics and Data Science at CMU [https://www.cmu.edu/dietrich/statistics-datascience/index.html] as a first-year PhD student this coming Fall 2022. For additional references mentioned in the show: * Quang's blog posts: https://qntkhvn.netlify.app/blog.html [https://qntkhvn.netlify.app/blog.html] * Code for paper: https://github.com/qntkhvn/climbing [https://github.com/qntkhvn/climbing] * Inducing Any Feasible Level of Correlation to Bivariate Data With Any Marginals [https://www.tandfonline.com/doi/full/10.1080/00031305.2017.1379438] * R copula package: https://cran.r-project.org/web/packages/copula/index.html [https://cran.r-project.org/web/packages/copula/index.html] and book: http://copula.r-forge.r-project.org/book/ [http://copula.r-forge.r-project.org/book/] * UConn Sports Analytics Symposium (UCSAS) [https://statds.org/events/ucsas2022/index.html] * CRAN Task View for Sports Analytics: https://cran.r-project.org/web/views/SportsAnalytics.html [https://cran.r-project.org/web/views/SportsAnalytics.html]
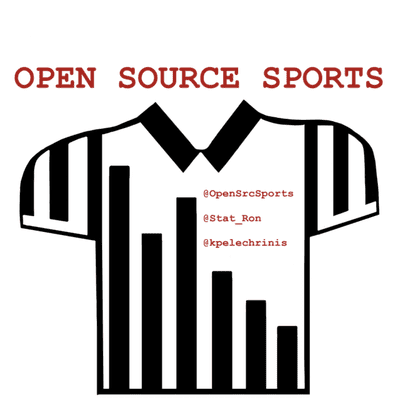
We discuss Grinding the Bayes: A Hierarchical Modeling Approach to Predicting the NFL Draft [http://stat.cmu.edu/cmsac/conference/2020/assets/pdf/Robinson.pdf] with Benjamin Robinson (@benj_robinson [https://twitter.com/benj_robinson]). This paper was a finalist in the Carnegie Mellon Sports Analytics Conference Reproducible Research Competition in October 2020. [http://stat.cmu.edu/cmsac/conference/2020/] You can submit an abstract to enter the 2021 Reproducible Research Competition [http://stat.cmu.edu/cmsac/conference/2021/] now! Benjamin Robinson is a data scientist living in Washington, D.C. and the creator of Grinding the Mocks, where since 2018 he has used mock drafts, the wisdom of crowds, and data science to predict the NFL Draft. He is a 2012 graduate of the University of Pittsburgh with degrees in Economics and Urban Studies and earned a Master of Public Policy degree from the University of Southern California in 2014. You can follow him on Twitter @benj_robinson [https://twitter.com/benj_robinson] and find the Grinding the Mocks project at grindingthemocks.com [https://grindingthemocks.com/] and @GrindingMocks [https://twitter.com/GrindingMocks]. For additional references mentioned in the show: * Ben's bitbucket repository of data: https://bitbucket.org/benjamin_robinson/grindingthebayes/ [https://bitbucket.org/benjamin_robinson/grindingthebayes/] * Bayesian modeling in R with the brms package: https://paul-buerkner.github.io/brms/ [https://paul-buerkner.github.io/brms/] * CMSAC Reproducible Competition abstract submission: http://stat.cmu.edu/cmsac/conference/2021/#mu-research [http://stat.cmu.edu/cmsac/conference/2021/#mu-research] * Saiem Gilani's (@SaiemGilani [https://twitter.com/SaiemGilani]) collection of software: https://sportsdataverse.org/ [https://sportsdataverse.org/]
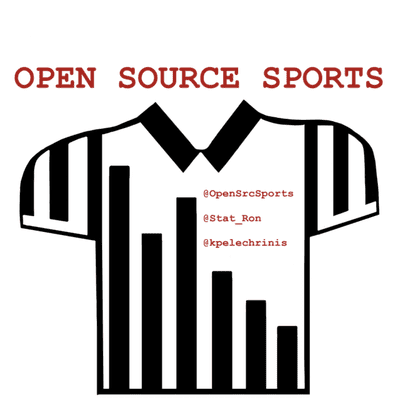
We discuss a previous Big Data Bowl [https://operations.nfl.com/gameday/analytics/big-data-bowl/] finalist paper `Expected Hypothetical Completion Probability` (https://arxiv.org/abs/1910.12337 [https://arxiv.org/abs/1910.12337]) with authors Sameer Deshpande (@skdeshpande91 [https://twitter.com/skdeshpande91]) and Kathy Evans (@CausalKathy [https://twitter.com/CausalKathy]). Sameer is a postdoctoral associate at MIT. Prior to that, he completed his Ph.D. at the Wharton School of the University of Pennsylvania. He is broadly interested in Bayesian methods and causal inference. He is a long-suffering but unapologetic fan of America's Team. He's also a fan of the Dallas Mavericks. Kathy is the Director of Strategic Research for the Toronto Raptors. She completed her Ph.D. in Biostatistics at Harvard University. She doesn't have an opinion on Frequentist vs Bayesian or R vs Python, but will get very upset if Rise of Skywalker is your favorite Star Wars movie. For additional references mentioned in the show: * Big Data Bowl notebooks: https://www.kaggle.com/c/nfl-big-data-bowl-2021/notebooks [https://www.kaggle.com/c/nfl-big-data-bowl-2021/notebooks] * BART: https://arxiv.org/abs/0806.3286 [https://arxiv.org/abs/0806.3286] * XBART: Accelerated Bayesian Additive Regression Trees https://jingyuhe.com/xbart.html [https://jingyuhe.com/xbart.html] * Matthew Reyers (@Stats_By_Matt [https://twitter.com/Stats_By_Matt]) thesis: https://twitter.com/Stats_By_Matt/status/1296570171687989249?s=20 [https://twitter.com/Stats_By_Matt/status/1296570171687989249?s=20]
Empieza 30 días de prueba
4,99 € / mes después de la prueba.Cancela cuando quieras.
Podcasts exclusivos
Sin anuncios
Podcast gratuitos
Audiolibros
20 horas / mes