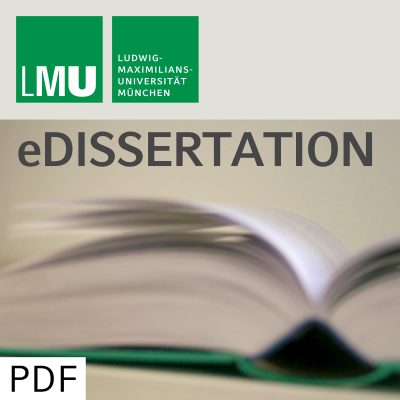
Fakultät für Mathematik, Informatik und Statistik - Digitale Hochschulschriften der LMU - Teil 01/02
Podcast von Ludwig-Maximilians-Universität München
Nimm diesen Podcast mit
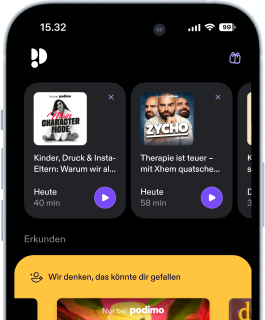
Mehr als 1 Million Hörer*innen
Du wirst Podimo lieben und damit bist du nicht allein
Mit 4,7 Sternen im App Store bewertet
Alle Folgen
250 Folgen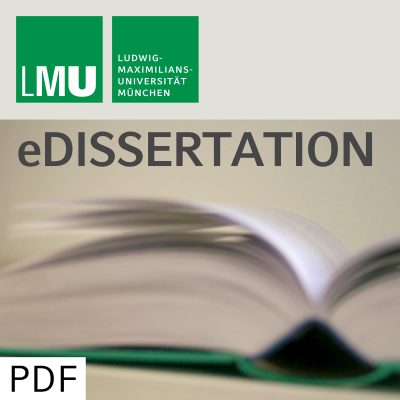
This thesis is concerned with the generalisation of Bayesian inference towards the use of imprecise or interval probability, with a focus on model behaviour in case of prior-data conflict. Bayesian inference is one of the main approaches to statistical inference. It requires to express (subjective) knowledge on the parameter(s) of interest not incorporated in the data by a so-called prior distribution. All inferences are then based on the so-called posterior distribution, the subsumption of prior knowledge and the information in the data calculated via Bayes' Rule. The adequate choice of priors has always been an intensive matter of debate in the Bayesian literature. While a considerable part of the literature is concerned with so-called non-informative priors aiming to eliminate (or, at least, to standardise) the influence of priors on posterior inferences, inclusion of specific prior information into the model may be necessary if data are scarce, or do not contain much information about the parameter(s) of interest; also, shrinkage estimators, common in frequentist approaches, can be considered as Bayesian estimators based on informative priors. When substantial information is used to elicit the prior distribution through, e.g, an expert's assessment, and the sample size is not large enough to eliminate the influence of the prior, prior-data conflict can occur, i.e., information from outlier-free data suggests parameter values which are surprising from the viewpoint of prior information, and it may not be clear whether the prior specifications or the integrity of the data collecting method (the measurement procedure could, e.g., be systematically biased) should be questioned. In any case, such a conflict should be reflected in the posterior, leading to very cautious inferences, and most statisticians would thus expect to observe, e.g., wider credibility intervals for parameters in case of prior-data conflict. However, at least when modelling is based on conjugate priors, prior-data conflict is in most cases completely averaged out, giving a false certainty in posterior inferences. Here, imprecise or interval probability methods offer sound strategies to counter this issue, by mapping parameter uncertainty over sets of priors resp. posteriors instead of over single distributions. This approach is supported by recent research in economics, risk analysis and artificial intelligence, corroborating the multi-dimensional nature of uncertainty and concluding that standard probability theory as founded on Kolmogorov's or de Finetti's framework may be too restrictive, being appropriate only for describing one dimension, namely ideal stochastic phenomena. The thesis studies how to efficiently describe sets of priors in the setting of samples from an exponential family. Models are developed that offer enough flexibility to express a wide range of (partial) prior information, give reasonably cautious inferences in case of prior-data conflict while resulting in more precise inferences when prior and data agree well, and still remain easily tractable in order to be useful for statistical practice. Applications in various areas, e.g. common-cause failure modeling and Bayesian linear regression, are explored, and the developed approach is compared to other imprecise probability models.
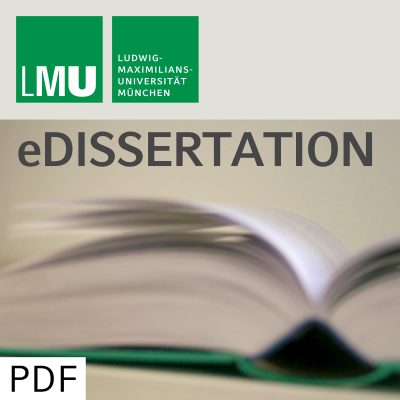
In this work local behavior for solutions to the inhomogeneous p-Laplace in divergence form and its parabolic version are studied. It is parabolic and non-linear generalization of the Calderon-Zygmund theory for the Laplace operator. I.e. the borderline case BMO is studied. The two main results are local BMO and Hoelder estimates for the inhomogenious p-Laplace and the parabolic p-Laplace system. An adaption of some estimates to fluid mechanics, namely on the p-Stokes equation are also proven. The p-Stokes system is a very important physical model for so-called non Newtonian fluids (e.g. blood). For this system BMO and Hoelder estimates are proven in the stationary 2-dimensional case.
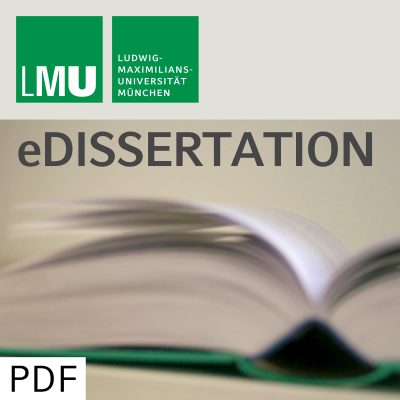
Dienstleister aus dem Bereich der Informationstechnologie (IT) stehen vor der großen Herausforderung, immer komplexere IT-Dienste kostengünstig anzubieten und diese effizient zu betreiben. Um dies zu erzielen, führt die Disziplin des IT-Service-Management (ITSM) strukturierte Managementprozesse ein. Werkzeuge unterstützen diese und stellen eine wichtige Schnittstelle zwischen Mensch, Prozess und Technik dar. Mit diesen Werk- zeugen lassen sich Prozesse koordinieren, die Technik effizient verwalten und wichtige Informationen für den Betrieb zusammenzuführen. Der geeignete Einsatz von Werkzeugen ist eine wesentliche Voraussetzung, um komplexe Aufgaben mit möglichst geringem Aufwand durchzuführen. Effizientes ITSM verfolgt somit auch stets das Ziel, Werkzeuge optimal einzusetzen und die ITSM-Prozesse sinnvoll zu unterstützen. Im Rahmen der Arbeit wird ein Ansatz vorgestellt, um den Einsatz von Werkzeugen entsprechend zu optimieren. Kern des Lösungsansatzes ist die Definition eines Reifegradmodells für Werkzeuglandschaften. Mit diesem lassen sich Werkzeuglandschaften begutachten und die Unterstützung der ITSM-Prozesse systematisch bewerten. Das Resultat ist eine gewichtete Liste mit Anforderungen an die Werkzeuglandschaft, um eine möglichst gute Prozessunterstützung zu erreichen. Aufgrund der Priorisierung der Anforderungen ist ein IT-Dienstleister nicht gezwungen, die Werkzeuglandschaft komplett in einem großen Schritt anzupassen. Stattdessen können die Verbesserungen sukzessive vorgenommen werden. Das Reifegradmodell unterstützt systematisch dabei, zunächst die wichtigsten Anforderungen umzusetzen, so dass die ITSM-Prozesse effektiv arbeiten können. Die Steigerung der Effizienz erfolgt dann in weiteren Schritten, indem zusätzliche Anforderungen umgesetzt werden. Die Erstellung eines solchen Reifegradmodells wird im Folgenden beschrieben. Zunächst wurden Anforderungen an einen geeigneten Lösungsansatz analysiert und ein Konzept für ein Reifegradmodell erarbeitet. Darauf aufbauend ist dieses Konzept beispielhaft angewendet worden, um ein Reifegradmodell für Werkzeuglandschaften zur Unterstützung von Prozessen nach ISO/IEC 20000 zu entwickeln. Die Arbeit schließt mit einer Evaluation des Lösungsansatzes ab, wobei das entwickelte Reifegradmodell empirisch in einem Szenario eines IT-Dienstleisters angewendet wurde. Mit der vorliegenden Arbeit wird die Grundlage für ein ganzheitliches und integriertes Management der Werkzeuglandschaft von IT-Dienstleistern geschaffen. Künftige Arbeiten können diese Methodik für spezifische Anwendungsszenarien übernehmen. Langfristig soll diese Arbeit als Grundlage dienen, um ein standardisiertes Reifegradmodell für Werkzeuglandschaften im Kontext von ITSM zu etablieren.
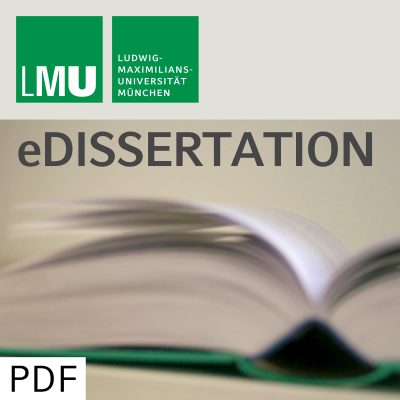
Both the current trends in technology such as smart phones, general mobile devices, stationary sensors and satellites as well as a new user mentality of utilizing this technology to voluntarily share information produce a huge flood of geo-spatial and geo-spatio-temporal data. This data flood provides a tremendous potential of discovering new and possibly useful knowledge. In addition to the fact that measurements are imprecise, due to the physical limitation of the devices, some form of interpolation is needed in-between discrete time instances. From a complementary perspective - to reduce the communication and bandwidth utilization, along with the storage requirements, often the data is subjected to a reduction, thereby eliminating some of the known/recorded values. These issues introduce the notion of uncertainty in the context of spatio-temporal data management - an aspect raising an imminent need for scalable and flexible data management. The main scope of this thesis is to develop effective and efficient techniques for similarity search and data mining in uncertain spatial and spatio-temporal data. In a plethora of research fields and industrial applications, these techniques can substantially improve decision making, minimize risk and unearth valuable insights that would otherwise remain hidden. The challenge of effectiveness in uncertain data is to correctly determine the set of possible results, each associated with the correct probability of being a result, in order to give a user a confidence about the returned results. The contrary challenge of efficiency, is to compute these result and corresponding probabilities in an efficient manner, allowing for reasonable querying and mining times, even for large uncertain databases. The paradigm used to master both challenges, is to identify a small set of equivalent classes of possible worlds, such that members of the same class can be treated as equivalent in the context of a given query predicate or data mining task. In the scope of this work, this paradigm will be formally defined, and applied to the most prominent classes of spatial queries on uncertain data, including range queries, k-nearest neighbor queries, ranking queries and reverse k-nearest neighbor queries. For this purpose, new spatial and probabilistic pruning approaches are developed to further speed up query processing. Furthermore, the proposed paradigm allows to develop the first efficient solution for the problem of frequent co-location mining on uncertain data. Special emphasis is taken on the temporal aspect of applications using modern data collection technologies. While the aforementioned techniques work well for single points of time, the prediction of query results over time remains a challenge. This thesis fills this gap by modeling an uncertain spatio-temporal object as a stochastic process, and by applying the above paradigm to efficiently query, index and mine historical spatio-temporal data.
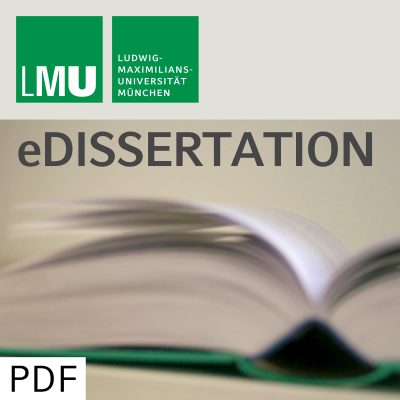
Relational learning is concerned with learning from data where information is primarily represented in form of relations between entities. In recent years, this branch of machine learning has become increasingly important, as relational data is generated in an unprecedented amount and has become ubiquitous in many fields of application such as bioinformatics, artificial intelligence and social network analysis. However, relational learning is a very challenging task, due to the network structure and the high dimensionality of relational data. In this thesis we propose that tensor factorization can be the basis for scalable solutions for learning from relational data and present novel tensor factorization algorithms that are particularly suited for this task. In the first part of the thesis, we present the RESCAL model -- a novel tensor factorization for relational learning -- and discuss its capabilities for exploiting the idiosyncratic properties of relational data. In particular, we show that, unlike existing tensor factorizations, our proposed method is capable of exploiting contextual information that is more distant in the relational graph. Furthermore, we present an efficient algorithm for computing the factorization. We show that our method achieves better or on-par results on common benchmark data sets, when compared to current state-of-the-art relational learning methods, while being significantly faster to compute. In the second part of the thesis, we focus on large-scale relational learning and its applications to Linked Data. By exploiting the inherent sparsity of relational data, an efficient computation of RESCAL can scale up to the size of large knowledge bases, consisting of millions of entities, hundreds of relations and billions of known facts. We show this analytically via a thorough analysis of the runtime and memory complexity of the algorithm as well as experimentally via the factorization of the YAGO2 core ontology and the prediction of relationships in this large knowledge base on a single desktop computer. Furthermore, we derive a new procedure to reduce the runtime complexity for regularized factorizations from O(r^5) to O(r^3) -- where r denotes the number of latent components of the factorization -- by exploiting special properties of the factorization. We also present an efficient method for including attributes of entities in the factorization through a novel coupled tensor-matrix factorization. Experimentally, we show that RESCAL allows us to approach several relational learning tasks that are important to Linked Data. In the third part of this thesis, we focus on the theoretical analysis of learning with tensor factorizations. Although tensor factorizations have become increasingly popular for solving machine learning tasks on various forms of structured data, there exist only very few theoretical results on the generalization abilities of these methods. Here, we present the first known generalization error bounds for tensor factorizations. To derive these bounds, we extend known bounds for matrix factorizations to the tensor case. Furthermore, we analyze how these bounds behave for learning on over- and understructured representations, for instance, when matrix factorizations are applied to tensor data. In the course of deriving generalization bounds, we also discuss the tensor product as a principled way to represent structured data in vector spaces for machine learning tasks. In addition, we evaluate our theoretical discussion with experiments on synthetic data, which support our analysis.